The YouTube recommendation system serves as a cornerstone of the platform’s user engagement strategy, shaping the viewing experience for millions worldwide. By analyzing user behavior, preferences, and interaction data, YouTube personalizes content suggestions to keep viewers entertained and engaged for longer sessions. The system’s sophisticated algorithms use a mix of user history, video metadata, and contextual information to introduce viewers to a diverse array of videos that are likely to catch their interest, ranging from popular trends to niche topics that align with individual tastes.
Understanding the intricacies of the recommendation engine is crucial for content creators aiming to optimize their visibility and for discerning viewers who wish to curate their digital environment. The YouTube algorithm has evolved to prioritize not only relevance and personalization but also responsible recommendations that reflect a commitment to fostering a diverse and inclusive platform. For users and creators alike, navigating the recommendation controls can lead to a more tailored and enjoyable YouTube experience, potentially impacting the broader digital culture and society’s consumption patterns.
Key Takeaways
- Our exploration provides insight into how YouTube’s recommendation system personalizes the user experience.
- We uncover the significance of diverse and responsible content curation for community engagement on the platform.
- Our analysis highlights the importance of the recommendation system in YouTube’s business and technological strategies.
Understanding YouTube’s Recommendation System
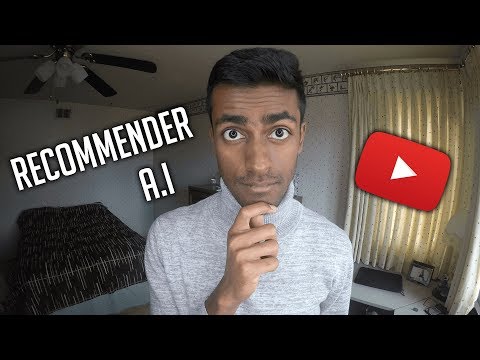
In our examination of YouTube’s recommendation system, we focus on its reliance on advanced algorithms and data processing through machine learning techniques. These technologies shape the personalized content that users encounter, impacting their viewing experiences significantly.
Algorithm Foundations
YouTube’s recommendation system is designed to serve users with videos they are likely to watch and enjoy. At its core, the system is powered by a complex algorithm that analyzes various signals. It includes user interaction history, such as videos watched, liked, and shared, as well actively measuring the time spent watching a video. The goal of this algorithm is not only to increase watch time on individual videos but also to enhance overall user engagement on the platform.
- User Data: Every interaction is used to understand preferences.
- Video Data: Details like video title, description, and performance metrics.
- Engagement Signals: Including likes, shares, and comments.
This multifaceted approach means that the YouTube recommendation system continuously evolves, showing users content that aligns with their interests.
Data and Machine Learning
The fuel for YouTube’s recommendation algorithm is the vast amount of data generated by over two billion logged-in users monthly. Machine learning algorithms are employed to parse this data, recognize patterns, and predict which videos will be most relevant to a specific user.
- Data Collection: Capturing engagement and video metadata.
- Pattern Recognition: Identifying user preference trends.
- Predictive Modeling: Suggesting future content a user is likely to enjoy.
By implementing machine learning, YouTube is capable of adapting recommendations in real-time, refining user experience as more data is collected. It’s a sophisticated process that necessitates the analysis of user feedback and behavioral changes to recommend content that not only interests users but may also expand their horizons.
Factors Influencing Video Recommendations

Before we delve into the specifics, it’s vital to understand that the YouTube recommendation algorithm focuses on personalization by interpreting various signals related to user interactions and content relevance.
User Engagement Signals
Engagement signals are data points that convey how viewers interact with content. These include likes, dislikes, the number of clicks, and watch time. A high number of likes indicates to YouTube’s algorithm that a viewer found a video useful, prompting it to recommend similar content. Conversely, dislikes signal a lower preference, impacting future recommendations. Watch history and search history also play crucial roles, as they directly reflect a user’s interests and viewing habits, leading to more personalized video suggestions.
- Likes/Dislikes: Impact video ranking in recommendations.
- Watch Time: Indicates video quality and viewer interest.
- Clicks: Reflect user interest and satisfaction with video suggestions.
Viewer Feedback
Feedback from viewers is instrumental in shaping recommendations. Direct feedback such as comments and survey responses give us tangible insights into viewer preferences. Moreover, viewing habits, such as whether a viewer finishes a video or leaves midway, offer indirect feedback that guides our system. The algorithm interprets this along with direct feedback to better match future video recommendations to user preferences.
- Comments: Provide qualitative insight into user engagement.
- Surveys: Directly ask viewers about their preferences.
- Viewing Habits: Offer clues on content relevancy to the viewer.
Content Analysis
Our system analyzes video content to understand its substance and relevance to the viewer. It looks at elements like captions, thumbnails, and video descriptions to categorize and recommend content effectively. This analysis is intertwined with the viewer’s search history and previously watched content to offer recommendations that align with known interests and potentially expand a viewer’s horizons.
- Video Descriptions: Help to categorize and recommend videos.
- Closed Captions: Allow content to be more accessible and analyzable.
- Thumbnails: Serve as the first impression and influence click-through rate.
Improving User Experience
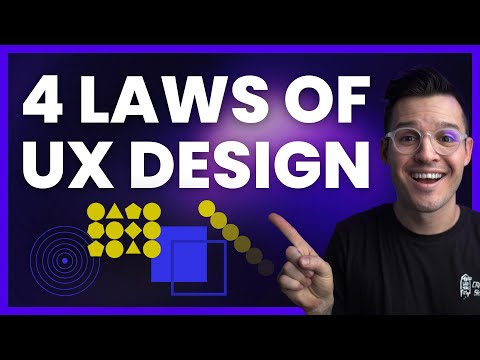
In our pursuit to enhance viewer satisfaction on YouTube, we focus on tactics that tailor user experience through Personalized Recommendations, gather actionable insights via Satisfaction Surveys, and adhere to Responsible Recommendation Practices.
Personalized Recommendations
We employ algorithms that analyze viewing habits to provide users with video suggestions that are closely aligned with their preferences, thus fostering a sense of time well spent on the platform. Personalized recommendations are a cornerstone of our strategy to keep users engaged and satisfied by delivering content that resonates with their interests.
Satisfaction Surveys
To further refine our understanding of user preferences, we implement satisfaction surveys that allow viewers to provide immediate feedback on the videos recommended to them. The survey responses are instrumental in assessing whether the content we present meets the individual expectations of our users.
Responsible Recommendation Practices
We recognize our responsibility as a platform to promote a positive and safe user experience. Our team is dedicated to ensuring that the recommendation system takes into account the broader impact on the community, striving to maintain a balance between personalized content and the overall health of the information ecosystem on YouTube.
Navigating Recommendation Controls
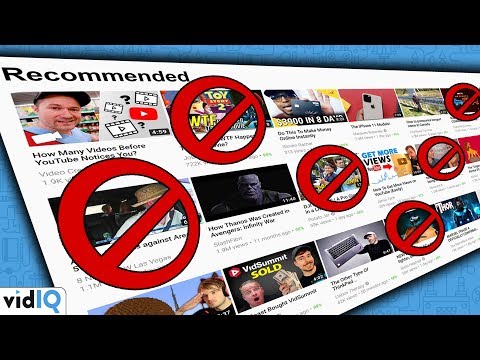
When we explore YouTube, understanding the recommendation controls helps us tailor our content feed. These controls empower us to influence the “Up Next” recommendations and adjust the content tailored to our preferences.
User Interface Options
Within YouTube’s interface, we find a variety of options that allow us to shape our viewing experience. If we encounter a video in our “Up Next” panel that doesn’t interest us, we can click “Not Interested” to remove it from our recommendations. We can also manage our preferences more extensively through the Settings menu, where we have the ability to turn off autoplay for recommended videos, providing us with a more hands-on approach to navigating recommended content.
Incognito Mode
For those times when we wish to browse without influencing our recommendations, YouTube offers an Incognito Mode. By engaging this mode, our activity, such as videos watched or searched for, won’t affect our future recommendations. It’s particularly useful when we’re exploring content that we don’t want to factor into our personalized suggestions.
Feedback Mechanisms
YouTube also gives us the opportunity to directly influence our recommendations through feedback mechanisms. By clicking the three dots next to a video title and selecting “Not Interested,” we can immediately remove a video from our recommendations. Additionally, we have the option to provide a reason for this choice, giving YouTube further insight to refine our future recommendations. Sharing this kind of feedback actively shapes our “Up Next” suggestions to better align with our interests.
The Impact of Community and User Actions
Before delving into specifics, it’s important to note that community guidelines play a crucial role in shaping the recommendation system on YouTube. They are enforced by both algorithmic measures and human evaluators. User interactions, on the other hand, like channel subscriptions, likes, and dislikes, signal individual preferences, influencing the system’s content curation.
Community Guidelines and Compliance
Our platform’s health and integrity largely hinge on the enforcement of Community Guidelines. These rules serve as a foundation for acceptable content and are assessed by a blend of advanced algorithms and dedicated human evaluators.
- Human Evaluators: Key enforcers who audit content to ensure guidelines are met.
- Algorithms: Automatic systems that filter large volumes of content.
Content breaching these guidelines is less likely to be recommended, protecting our users from harmful or deceptive material.
User Interactions
Every click, like, dislike, and subscription a user makes feeds into the YouTube algorithm, reflecting individual content preferences.
- Likes/Dislikes: Act as direct feedback on video relevance and quality.
- Channel Subscriptions: Indicate a user’s interest in recurring content from specific creators.
These interactions fine-tune the recommendation system, aiming to increase user satisfaction and engagement by delivering more personalized content streams.
Evolving with Technology and Society
As technology advances, the YouTube recommendation system must adapt, addressing both the power of machine learning and the spread of misinformation.
Machine Learning Advancements
We have witnessed significant advancements in machine learning, which have immensely impacted YouTube’s recommendation algorithm. This technology enables YouTube to curate content tailored to individual user preferences. The platform continuously integrates complex models to understand user interactions, improving accuracy in video suggestions. A pertinent example is YouTube’s handling of borderline content. Aimed at reducing the reach of such videos, YouTube employs machine learning to identify and limit recommendations for content that comes close to—but does not quite breach—its community guidelines.
Addressing Misinformation
Tackling misinformation remains a critical challenge for us as a responsible platform. We prioritize the recommendation of authoritative videos to provide reliable information to our users. For controversial topics, such as those claiming the earth is flat, we’ve adjusted our algorithms to promote content from credible sources. It’s part of our commitment to ensure that the evolution of our recommendation system aligns with societal values and the quest for factual integrity.
Maintaining a Diverse and Inclusive Platform
We understand the critical role that diverse content plays in fostering an inclusive platform. Ensuring that a variety of quality information reaches different audiences is central to our approach.
Encouraging Varied Content
We actively work to encourage content creators to produce varied material that reflects the wealth of diversity that our audience embodies. By implementing sophisticated filters and monitoring systems, we strive to balance viewership across a spectrum of voices and perspectives. This approach not only enriches the viewer experience but also promotes a culture of inclusion. Whether a creator is covering niche topics or engaging with mainstream issues, our goal is to provide equal opportunities for visibility and success on our platform.
It’s our belief that a platform thrives when diverse ideas and cultures are given a stage. With each decision, we aim to filter through noise, foregrounding quality information and bringing forward narratives that might otherwise go unheard. Through this, we build a richer, more varied viewing experience for our audience.
Insight into YouTube’s Business Strategy
We must understand that YouTube’s business strategy intricately balances viewership growth with a commitment to social responsibility. Their approach includes optimizing the vast amount of content, including music videos, to cater to user preferences while maintaining a platform that supports creators and advertisers alike.
Balancing Viewership and Responsibility
We prioritize creating an environment that fosters viewership and engagement on YouTube. This entails not only tailoring recommendations to individual users but also ensuring these recommendations maintain our commitment to responsible content curation. By promoting videos from a variety of subscribed channels, we aim to empower users with diverse and fresh content that keeps them returning to the platform.
Supporting Creators and Advertisers
We recognize our role in facilitating a symbiotic relationship between creators and advertisers. By providing tools for creators to optimize their content for better visibility, we bolster their ability to attract advertising partnerships. Simultaneously, we aid advertisers in reaching their desired audience through targeted campaigns, which include prominent placement in music videos and other high-traffic areas on YouTube, strengthening the economic framework of our platform.